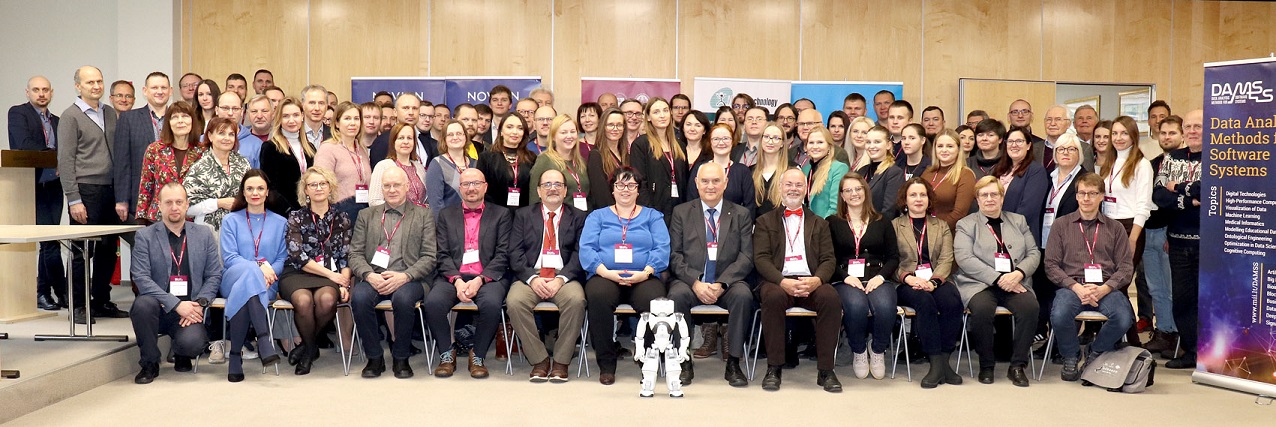
The DAMSS 2022 programme.
Download
DAMSS 2022: Plenary Speakers
![]() |
Prof. Konstantinos Diamantaras
Konstantinos Diamantaras received the Diploma degree from the National Technical University of Athens, Greece, and the Ph.D. degree in Electrical Engineering from Princeton University, Princeton, NJ, USA, in 1992. He joined the Department of Information Technology, in the TEI of Thessaloniki, Greece as a faculty member in 1998. He is currently a Professor at the Department of Information and Electronics Engineering, International Hellenic University, Greece. His research interests include machine learning, signal / image processing and parallel computing. Dr. Diamantaras has served as the Chairman to the Machine Learning for Signal Processing (MLSP) Technical Committee (TC) of the IEEE Signal Processing Society and a member of the MLSP and Signal Processing Theory and Methods TCs as well. He has been the Chairman and a member of the TC for various machine learning, signal processing, and neural networks conferences. He has served as an Associate Editor for the IEEE Transactions on Signal Processing, the IEEE Signal Processing Letters, and the IEEE Transactions on Neural Networks. |
Talk title: Natural Language Generation problems
Abstract: Natural Language Generation problems like question-answering, text summarization, and machine translation are nowadays tackled using mainly transformer-based machine learning models such as GPT-3, T5, and BART. Although these sophisticated models achieve very good performance in most NLG tasks, they suffer from a fundamental lack of common sense. For this reason, they often generate implausible and "strange" sentences or sentences that are short and simple, avoiding the rich and natural structures generated by humans. Recently there is an increasing trend to incorporate commonsense reasoning in text generation. The aim is to enhance/enrich the process of natural language generation using external knowledge which exists in many data sources like Wikipedia, knowledge bases, and knowledge graphs. Common-sense knowledge is one example of knowledge that can be acquired from knowledge bases/graphs and can be used in the generation process by creating embeddings. The focus of this talk is to present methods that incorporate external knowledge that is available in various commonsense knowledge bases into state-of-the-art natural language generation models.
![]() |
Prof. Dr. Pasi Fränti
Pasi Fränti received his MSc and PhD degrees from the University of Turku, in 1991 and 1994 in Science. Since 2000, he has been a professor of Computer Science at the University of Eastern Finland. He has published in 99 journals and 175 peer review conference papers. Pasi Fränti is the head of the Machine Learning research group. His current research interests include clustering algorithms, location-based services, machine learning, web and text mining, and optimization of health care services. He has supervised 30 PhD graduates and is currently supervising nine more. |
Talk title: Clustering Healthcare Data
Abstract: Clustering can a powerful tool in analyzing healthcare data. We show how clustering based on k-means and its variants can be used to extract new insight from various data with the aim to better optimize the health care system. We first show that simple variants of k-means and random swap algorithms can provide highly accurate clustering results. We demonstrate how k-means can be applied to categorical data, sets, and graphs. We model health care records of individual patients as a set of diagnoses. These can be used to cluster patients, and also create co-occurence graph of diagnoses depending on how often the same pair of diseases are diagnosed in the record of the same patient. Taking into account the order of the diagnoses, we can construct a predictor for the likely forthcoming diseases. We also provide a clustering algorithm to optimize the location of health care systems based on patient locations. As a case study, we consider coronary heart disease patients and analyze in what way the optimization of the locations can affect the expected time to reach the hospital within the given time. All the results can provide additional statistical information to healthcare planners and also medical doctors at the operational level to guide their efforts to provide better healthcare services.
![]() |
Jevgenij Gamper
Jevgenij Gamper is a Staff Decision Scientist at Vinted. Together with the experimentation team Jev co-leads across all aspects of experimentation and causal inference. From implementing scientific models underlying the experimentation system to setting up technical roadmap and establishing experimentation culture. Prior to that Jev co-lead a machine learning team at Vinted. His past experience covers a wide spectrum of scientific modelling and leadership in industry and academia. From developing Gaussian process based exo-planetary validation algorithms for Kepler satellite data, to computer vision with remote sensing data and climate modelling in industry, as well as PhD research in medical imaging. Jev's PhD publications have appeared at top research venues such as CVPR, and are licensed to the pharmaceutical industry. Jev's passion is to bridge the gap between industry and academic research communities. |
Talk title: A deep dive into industry inspired research questions: from Causal Inference and Machine Learning, to Complexity Science
Abstract: In this talk I will motivate several open research directions inspired by real industry scenarios and constraints at Vinted. For each research direction, I will present a business case, its mathematical formalisation and derived open questions, prior literature, as well as avenues for developing answers to these scientific questions. The topics I will cover include but are not limited to: Causal discovery and experiment design; Machine learning and feedback loops; Complex system simulation; Machine learning, surrogacy and mediation analysis.
![]() |
Prof. Dr. Eligius M.T. Hendrix
Prof. Dr. Eligius M.T. Hendrix is a European scientist with more than 35 years of experience in mathematical modelling and optimization algorithms. His research focuses on exploiting the mathematical structure of optimization problems in order to derive novel specific algorithms that can be implemented on modern computer platforms. Most of his work was related to practical problems in environmental and food science. Among others, he developed a new method for unmixing data from hyperspectral data and is interested in data selection of training sets for Deep learning on those data from the point of view of the design of experiments. Moreover, his studies enhance logistics, inventory control, competitive location problems, production scheduling, traffic control, minimizing the size of search trees, fisheries quota determination, offshore wind farm maintenance, pooling, water control, food supply chains, coalition formation, deforestation, economic behaviour, design of experiments, permit trading, biomass production, fodder production, farm management and plague control. He published more than 85 journal articles and several books and organized international conferences such as Global Optimization workshops and ICCSA. He is affiliated with the Universidad de Málaga. |
Talk title: On Global Optimization and Machine Learning
Abstract: Machine Learning of predictive and classification models can be viewed from an optimization perspective. Stochastic gradient approaches implemented in learning algorithms may suffer from effective convergence pushing researchers to go for random approaches based on a randomly selected training set of data. We focus on the characterization of the underlying optimization landscape and pose some questions on the effectiveness of algorithms. We focus on parameter estimation, ill-conditioning, parameter identification and symmetry leading to infinitely many parametrizations providing similar performance, also called over-parametrization in deep learning. Attention is paid to using the design of experiments to select the training data set. We use several small instances to showcase the underlying difficulties.
![]() |
Prof. Dr. Audronė Jakaitienė
Audronė Jakaitienė is a Professor and Chief Researcher at Vilnius University. Prior to this, she held a position as Senior Economist at the Bank of Lithuania. She has also worked as Senior Expert (Economist) for the European Central Bank. Prof. A. Jakaitienė is a Board member of the Lithuanian Statistical Society; Lithuania’s representative at the International Biometric Society. From 2019 she is a member of the European Statistical Advisory Committee (ESAC). She conducts research in econometrics, biostatistics, and statistics of education. |
Talk title: How much do we collect and how much do we use for policy and research? Education Data. Case of Lithuania
Abstract: We will review various sources of educational data (e.g., international large-scale studies, data registers) and their use for policy decisions and research in Lithuania. It has been shown that a lot of data has already been collected and that more is being accumulated. Up to 20 percent of the information gathered is used for policy purposes and even less in research. It is noted that most of the data collected is useful for the economic paradigm. We will present a case study demonstrating that national population-based studies and international achievement studies can send different messages and cannot be considered in isolation.
![]() |
Dr. Giovanna D’Inverno
Giovanna D'Inverno is a Tenure-track Assistant Professor in Mathematical Methods for Economics, Actuarial Science and Finance at the Department of Economics and Management, University of Pisa (Italy). She has a joint PhD degree (2018) from Scuola IMT Alti Studi Lucca (Italy) and KU Leuven (Belgium), a postdoc at KU Leuven in the Leuven Economics of Education Research center and a flourishing academic career in the field of quantitative methods and public economics, with publications in top-ranked journals. Her research focuses on the development of quantitative methods and policy evaluation techniques to address policy-relevant issues, so as to complement efficiency with effectiveness analysis in an integrated economic framework. Recurrent empirical applications mostly cover public economic issues and sustainability challenges, with particular attention to education and public economics. She works on several national and international projects, including collaborations with consultancy agencies and policy makers. |
Talk title: All for one and one for all: How to assess performance when there are different dimensions and different stakeholders’ priorities?
Abstract: : Composite indicators are often used to aggregate several dimensions in one single score, so to provide an overall performance measure. In the evaluation process, there are key elements that need to be considered. First, it is important to measure whether and to what extent set performance targets are met. Second, the aggregation must reflect and harmonize the different preferences of the involved stakeholders. In this talk, we discuss a new composite indicator that integrates the Goal Programming Synthetic Indicator methodology with the Analytic Hierarchy Process. We showcase its potential by evaluating to which extent European countries fulfil the European Union requirements in terms of municipal waste management while taking into account preferences as expressed by a panel of experts.
![]() |
Dr. Dmitry Podkopaev
Dmitry Podkopaev received the Ph.D. degree in mathematics and physics from the Institute of Mathematics, National Academy of Sciences of Belarus (1999), and the title of Docent in Multiobjective Optimization from the Department of Mathematical Information Technology of the University of Jyväskylä, Finland (2014). He currently works as an Assistant Professor at the Systems Research Institute, Polish Academy of Sciences, and participates in two research projects on supply chain optimization. His main research interests include multiobjective and combinatorial optimization as methodologies for decision making in complex systems. He develops optimization methods, preference models, and interactive decision support systems, and has applied multiobjective optimization methods in various application domains, such as medicine, finance, computer systems, and supply chain management. |
Talk title: Decision support for many-objective optimization.
Abstract: The abundance of data and the development of information technologies enable solving large-scale decision-making problems at high levels of detail. In some applications, it is reasonable to consider many individual objectives to accurately address the balance of interests. Moreover, if multiple scenarios are introduced to model uncertainty, the number of objectives increases many-fold. However, dealing with many objectives using traditional decision-support tools is problematic due to the limitations of human cognitive capacities. We present recent developments of techniques that provide interactive decision support for solving many-objective optimization problems.
Publications